Drone imaging to evaluate impact of Nematodes on Soybean Yield
ID
BSE-362NP
Introduction
Globally, soybeans are one of the most important crops grown for food and feed. With the growing demand for food worldwide, prompt estimation of soybean yield (SY) is critical for growers to administer precision crop management decisions for optimal yield realization. Numerous stressors, most notably soybean cyst nematode (SCN) can drastically lower soybean yield (Arantes et al., 2021). The nematodes feed off the soybean roots initiating the infection. Traditionally, assessment of nematode infestation is done through manual scouting by looking at visible symptoms because of crop response. This method often requires specialists, is time consuming and the observed symptoms could be misdiagnosed with nutrient deficiencies, diseases as well as other pest attacks. In addition, laboratory-based root analysis as well as microscopic counting of nematodes from soil samples is required to identify the degree of infestation which is highly time consuming, and further delays obtaining results to implement control measures by the growers. Against these limitations, use of drone-based multispectral imaging is prompt and provides a potential solution for nematode detection. On the other hand, yield is generally recorded using yield monitors on combine harvesters at season end. These yield monitoring devices need regular field calibration, are intrusive, costly to own and run, and often require heavy data handling to extract meaningful information. This creates a need for timely interventions that are noninvasive, provide real time information with applicability over larger field sizes and eventually can help growers implement precision crop management practices such as fertilizers, pesticides, and irrigation applications as well as harvest and storage decisions (Jjagwe et al., 2023; Chandel et al., 2021).
Emerging non-invasive techniques
Remote sensing technologies from ground based (crop temperature sensors, soil moisture sensors, handheld spectrophotometer), satellite based (Landsat 8, Sentinel), and drone-based platforms have emerged for monitoring crop health, biomass, stressors (such as pests and diseases), as well as yield. Ground based devices are labor intensive and time consuming while satellite-based systems are affected by cloud covers and lower resolution or granularity (Jjagwe et al., 2023). Drones overcome these limitations due to their ease of covering large areas providing real time data on demand.
Drone imaging for nematode detection and yield estimation
Over the past 20 years, the use of drones (unmanned aerial vehicles) for agricultural operations has increased significantly particularly in crop pest and disease detection as well as yield estimation. Drones are equipped with spectral/imaging cameras that provide detailed information (up to mm/pixel) that can be used for informing accurate and prompt crop management decisions. This information provides insights into crop variations in the field which might not be possible with manual scouting or yield monitors. Furthermore, insights derived from drone data can be used to plan for future actions and crop planning such as irrigation, spraying, harvest, storage, and insurances, among others.
So far, drone imagery has been very limitedly explored for identifying crop responses and problems pertaining to nematodes in soybean production systems. This article attempts to address the gap using drone-based multispectral imagery and machine learning approach. Aerial imagery typically includes visible or RGB, multispectral, and hyperspectral ranges among others (Chandel et al., 2021). Vegetation indices (VIs) derived from spectral imagery such as the Normalized Difference VI (NDVI), Green-NDVI, and Normalized Difference Red-Edge Index (NDRE) are some of the most often used features for crop analysis. Usually, higher values of the VIs indicate higher yields while lower values indicate problems.
Machine Learning models
Machine learning models are mathematical algorithms that can be used to analyze and identify patterns and relationships in data. Their application in agriculture has grown over the past decade. Some of the most commonly used models include Random Forest (RF), and support vector machine (SVM), among others (Jjagwe et al., 2023). Machine learning models can estimate or extract valuable information on crop responses using input information which in this study are drone-based spectral and physiological features. Overall, this article summarizes recent exploration of Precision Agriculture Technologies and Data management program at Virginia Tech where impacts of nematode infestations on soybean is quantified non- intensively using drone-based multispectral imagery integrated with machine learning models.
Experimental study and data collection
Two soybean seed varieties, i.e., resistive and susceptible to parasitic nematodes, were planted in the 2022 growing season at the Tidewater Agriculture Research and Extension Center in Suffolk, VA (Fig. 1) by the plant pathology program. Each variety was planted in two-row plots, 30 feet length and spaced at 36 inches. Six replicate plots of nine fungicide treatments were planted that amounted to a total of 108 study plots. Ground data was collected at three crop stages: crop vigor in the early season (July 7 and 21, 2022), SCN counts in the late season (October 21, 2022), and yield at season-end (December 5, 2022). A drone (DJI Phantom 4 Multispectral) was flown over the field in mission mode near the late season of the crop (October 18, 2022) to collect multispectral images. The flight was conducted near solar (± 2h) noon period for high quality crop feature acquisition. Additionally, the drone has a skyward facing light sensor to measure the amount of solar radiation during flight. This measurement is crucial to adjust for spectral discrepancies brought on by variations in the amount of sunlight. VIs were then derived from acquired imagery data which were used to identify distinction in crop status as a result of nematode infestations. These VIs were then used inputs for the machine learning models (RF and SVM) for crop yield estimations. The estimated pre-harvest yield from the models was compared with the ground collected yield to evaluate the model performance using metrics of Pearson correlation (r) and relative root mean square error (rRMSE, %).
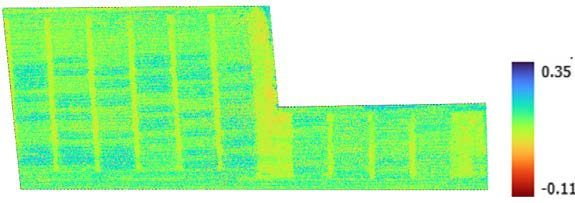
Results
Pearson correlation analysis (r) showed that GNDVI, (r = –0.6) and NDRE (r = –0.5) had good accuracy for showing differences of nematode population in the resistive and susceptible varieties of planted soybeans (Fig.2). Modified Soil Adjusted VI (MSAVI2, r = –0.37) had the lowest accuracy among all tested VIs. Higher nematode population was observed in the susceptible varieties (range = 20 to 1080) compared to the resistive varieties (range = 0 to 340). Fungicide treatments coupled with soybean cultivars also showed differences in nematode populations where some treatment combinations significantly lowered the nematode populations compared to others.
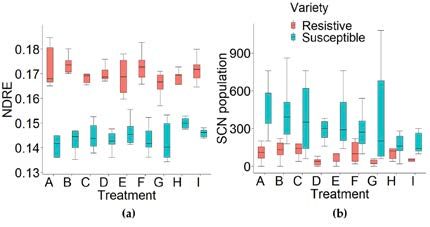
Pearson correlations (r) showed that NDRE (r = 0.75) had the strongest relationship with yield followed by GNDVI (r = 0.73), while Green Difference VI (r = 0.36) had the least strong relationship with yield. Yield was also observed to be significantly higher for the resistive variety (on average +15 lbs/plot) compared to susceptible variety (Fig. 3). Since some fungicide treatments (e.g., D & G) were able to control nematode populations, pertaining yields were amongst the highest recorded yields.
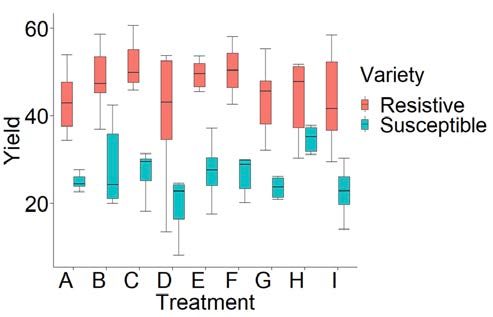
Random forest machine learning model showed the highest accuracy for soybean yield estimated much earlier in the season (r = 0.93, rRMSE =13.32%). Support vector machine model ranked second in terms of yield prediction accuracy (r = 0.82, rRMSE =21.39).
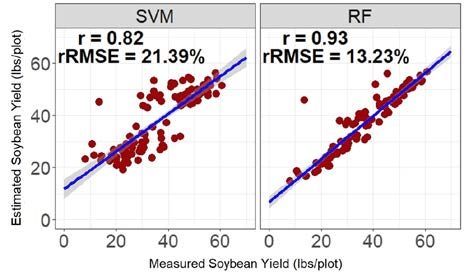
Conclusion
Drone based imagery shows the potential to highlight differences in crop status pertaining to nematode populations in soybean. In addition, integrating the imagery with machine learning models enables yield estimation much before harvest. This provides growers with insights into crop health and yield conditions prompting for timely management decisions such as application of fertilizer or fungicides and irrigation. In addition, growers would also benefit from preharvest yield estimations for harvest, storage, and sales planning. This helps to realize optimal yield. Our on-going efforts aim to use these findings to incorporate other environmental factors such as weather and soil to improve crop problem detection and yield estimation accuracies.
References
Arantes, B. H. T., Moraes, V. H., Geraldine, A. M., Alves, T. M., Albert, A. M., Silva, G. J. D., and G. Castoldi. 2021. Spectral detection of nematodes in soybean at flowering growth stage using unmanned aerial vehicles. Ciência Rural, 51, e20200283.
Chandel, A.K., L.R. Khot, and L.X. Yu. 2021. “Alfalfa (Medicago sativa L.) crop vigor and yield characterization using high-resolution aerial multispectral and thermal infrared imaging technique.” Computers and Electronics in Agriculture 182, p.105999.
Jjagwe, P., Chandel, A.K., and D. Langston. 2023. "Pre-Harvest Corn Grain Moisture Estimation Using Aerial Multispectral Imagery and Machine Learning Techniques." Land 12, 2188.
Virginia Cooperative Extension materials are available for public use, reprint, or citation without further permission, provided the use includes credit to the author and to Virginia Cooperative Extension, Virginia Tech, and Virginia State University.
Virginia Cooperative Extension is a partnership of Virginia Tech, Virginia State University, the U.S. Department of Agriculture, and local governments. Its programs and employment are open to all, regardless of age, color, disability, sex (including pregnancy), gender, gender identity, gender expression, genetic information, ethnicity or national origin, political affiliation, race, religion, sexual orientation, or military status, or any other basis protected by law.
Publication Date
November 7, 2024